When two different concepts sound or act alike, it can be easy to conflate them. We see this in everyday life: jelly vs. jam, weather vs. climate, latitude vs. longitude, concrete vs. cement, the list goes on. In the ever-expanding world of data jargon, this kind of problem can easily become prevalent.
Two modern concepts that succumb to this phenomenon are data mesh vs. data fabric. As these topics have gained popularity, they’ve become increasingly intertwined–and subsequently, are often confused for one another. In this blog, we’ll define each concept, explain their relevance, and compare and contrast data mesh vs. data fabric to help you make informed decisions about the architecture of your modern data stack.
What is a Data Mesh?
First defined by Zhamak Dehghani in 2019, data mesh provides an alternative to the monolithic architectures of many legacy data ecosystems. These older systems often centralized data ownership under select technical stakeholders and, in turn, bottlenecked data access and use due to manual processes.
Data mesh architectures offer an opposite approach, distributing responsibilities to a range of users to facilitate a more decentralized and accessible data ecosystem. This concept is organized around four core principles:
- Domain-Centric Ownership & Architecture: Domain ownership and management are shifted to teams that work most closely with and possess the most knowledge about each respective data domain.This ensures that the business team owning each domain collaborates with data consumers to facilitate timely and reliable data access.
- Data-as-a-Product: By considering data resources through a product lens, teams can adopt practices centered around ease of use. Ensuring data is good quality, documented, and discoverable means data users can access, share and leverage it more efficiently.
- Self-Service Data Platform: By implementing consistent domain-agnostic access and security measures that are low maintenance and easy-to-understand, teams create a data structure that is both clearly defined and repeatable across domains.
- Federated Computational Governance: With data readily available to so many users, maintaining consistent data governance and security measures across domains is an integral component of data mesh architectures. This makes global policies necessary in ensuring that data is consistently secured, regardless of which user is accessing it for which purposes.
Teams looking to democratize data use for stakeholders across their organization are beginning to implement data mesh architectures built on these principles. In doing so, they are driving enhanced data use and better business results across the board.
What is a Data Fabric?
Originating just a few years before data mesh, the concept of a data fabric was coined as an approach to end-to-end data unification for modern organizations. With the fast-moving development and adoption of new cloud data platforms on top of existing legacy tools, there is often a lack of cohesion in the data stack. Data fabric architectures are geared towards synthesis, bringing these disparate data resources, platforms, and tools together into a single “fabric” that unites and organizes them coherently.
While there’s no concrete model for this concept, any data fabric architecture should include capabilities such as:
- Ingestion & Discovery: This layer will ensure consistent new data discovery and integration into the fabric, making sure unification continues perpetually beyond the initial setup of the architecture.
- Integration & Abstraction: Bring data together from any/all lakes, warehouses, or other storage platforms, and abstract the information in order to eliminate complexity from the access layer.
- Unified Access & Querying: Allow users to query data from any and all sources in the ecosystem using one unified and abstracted data access layer.
- Data Security & Governance: When centralizing access to data resources, it is essential to include a data management layer that enforces effective data security and governance consistently across the ecosystem.
- Data Orchestration: With data coming in from such varied resources, it is crucial that the information is transformed and cleansed appropriately for consumption by the users who queried it.
Think of a hybrid data stack like the human body: your brain, stomach, lungs, and muscles each have independent purposes that they need to fulfill, just like data storage, compute, analysis, governance, and security platforms do. But as disparate parts of the larger system, each of these parts needs to communicate with the others to ensure the successful operation of the whole. Your body has the nervous system to take care of this task, connecting your brain to the rest of your organs to coordinate the right actions at the right times. The data fabric is designed to play this same role, integrating the data stack end-to-end to empower standardization and communication across the board.
Comparing Data Mesh vs. Data Fabric
With each concept defined, how can data mesh and data fabric be compared and contrasted in their applications?
Similarities
Starting simple, both data mesh and data fabric are frameworks/architectures through which data ecosystems can be organized. They are structural paradigms that aim to unify and streamline data use across disparate resources, teams, and purposes. Both have also arisen in response to the growing difficulty of keeping legacy data systems aligned in modern data environments.
In the pursuit of future-proof success, both data mesh and data fabric are designed to streamline and expedite data use at scale. Data ecosystems are not getting any smaller, and more tools and users only require more dynamic access and security capabilities. If a data architecture cannot scale with organizational growth, then it cannot be successful moving forward. Both mesh and fabric are built to enable continued growth as teams look to unlock more value from data, giving them easy access to–and causing them to be increasingly driven by–valuable data resources.
Differences
At their cores, data mesh and data fabric strive for enhanced data use from two different angles: decentralization vs. unification.
Data mesh structures data resources in a way that places users in charge of their respective domains. Providing users with self-service access to the data that they need to achieve their objectives is the core of a mesh framework. This is done to decentralize controls and avoid bottlenecks at the permissions level. Governance and security are federated across these distributed domains, and data use is left to the users.
Data fabric architectures, in contrast, are based on unification instead of distribution. While the end goal is still to give users self-service access to resources, it is done by weaving platforms, resources, and tools more closely together. By acting as the “nervous system” of the data ecosystem, the fabric unites all platforms and resources into a single cohesive framework that controls and secures access accordingly for users.
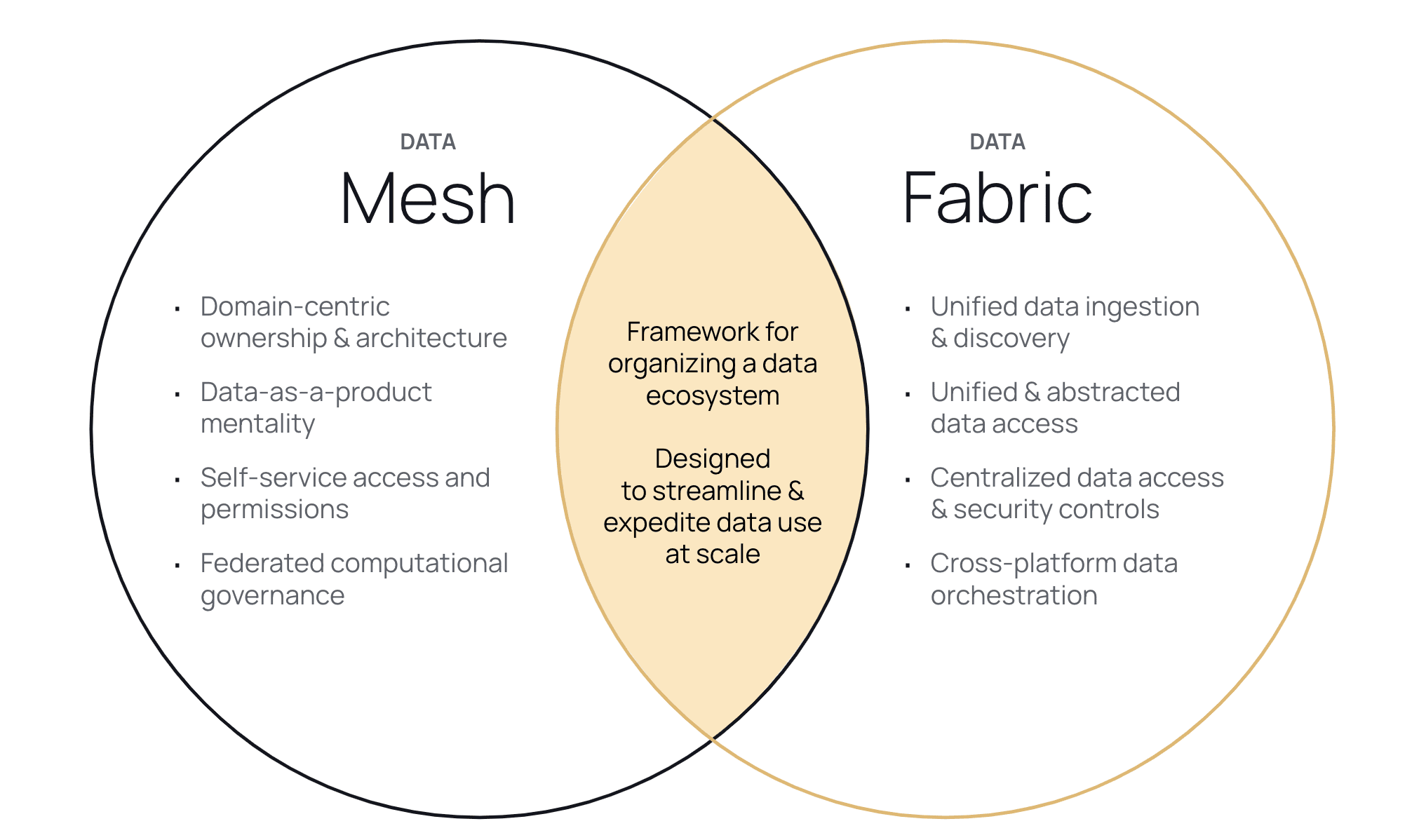
Securing Data Mesh vs. Data Fabric
Regardless of their differences, both data mesh and data fabric architectures emphasize the need for powerful data governance, access, and security capabilities. Without these, their respective approaches – decentralization and unification – would only increase the risk of putting the wrong data in the wrong hands.
Both of these architectures require data security that spans across platforms, domains, and users in a consistent manner. A dynamic data security platform solves this need for mesh and fabric frameworks, enabling teams to author and apply security policies once and have them automatically enforced on any user query. Data discovery and detection capabilities strengthen security even more by giving teams a holistic view of the resources they have, and how they are being accessed and used.
To see how the Immuta Data Security Platform enables modern data architectures, check out our eBook Powering Your Data Mesh with Snowflake & Immuta. You can also learn how Roche Diagnostics uses Immuta to empower their data mesh here.