We're moving from a deterministic world where we codify every potential use case, every potential end user, and what they could see, to a risk-based world where we have to make the best decision based on the context that we have in front of us as to who should access what data, when, where, why.”
Matthew Carroll, Co-Founder & CEO, Immuta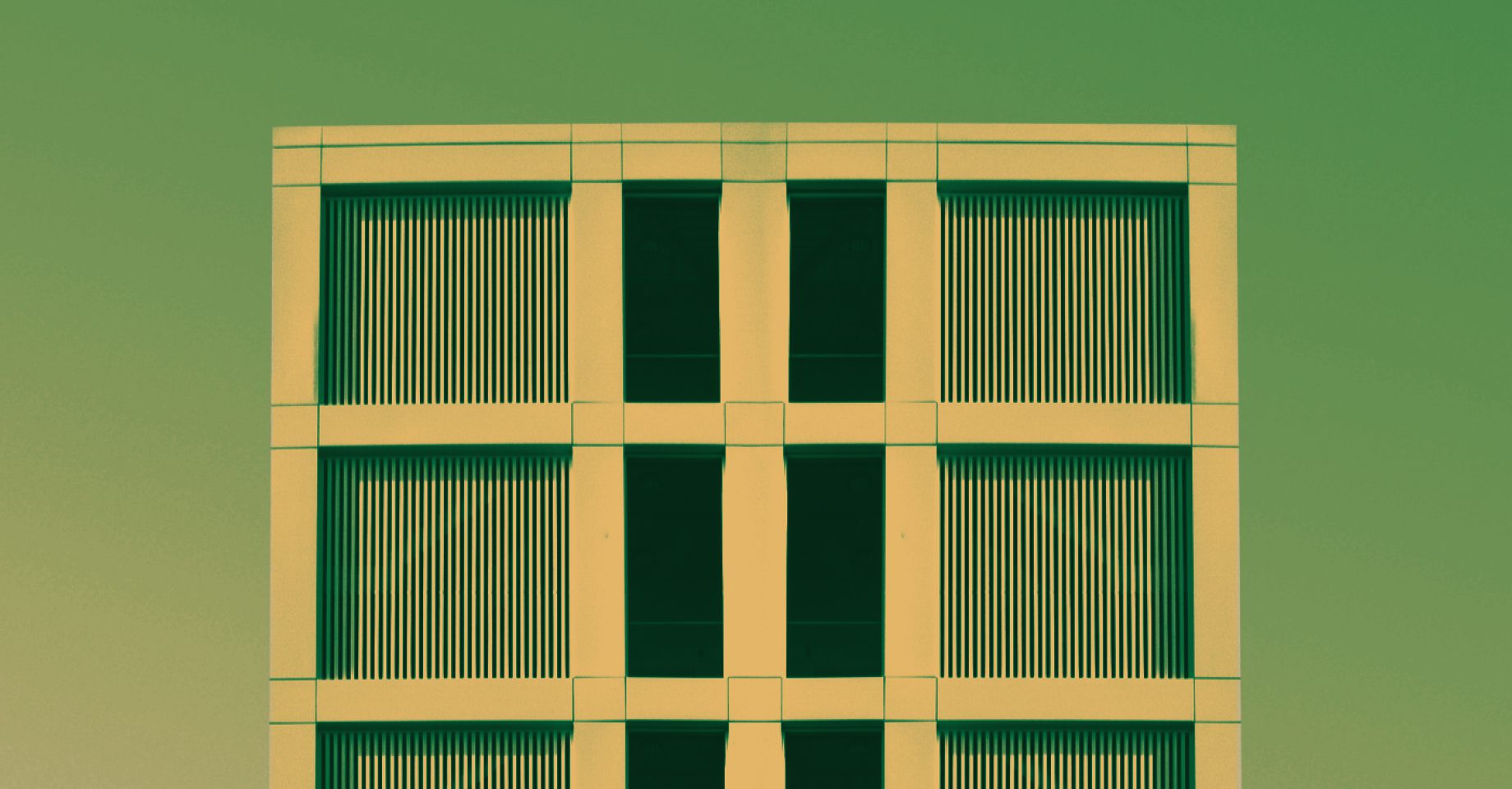
From artificial intelligence and machine learning advancements, to decentralized cloud storage and analysis, and other novel use cases, we’re seeing data innovations happening before our eyes. At the same time, data breaches and noncompliance penalties are increasingly common and costly, and threats to data will only continue to grow.
In this dynamic environment, traditional data security controls are no longer a viable defense. There is a necessary shift occurring – away from exhaustive, deterministic access controls and towards probabilistic, risk-aware policies. This adds a crucial layer of context to data security and privacy controls.
Immuta CEO Matt Carroll joined analyst Sanjeev Mohan’s It Depends podcast to discuss how these increasing demands on the modern data stack are driving a shift in data privacy and security – not just in how we apply controls, but in how we think about protecting sensitive data at large. Here, we’ll highlight the key points of their conversation and uncover why risk-aware policies are central to the future of data use.
The Legacy of Deterministic Data Access and Security
Securing data access has traditionally been a straightforward, deterministic process. Predetermined policies restrict access to stored data and when a user wants to see that data, an administrator or policy either grants or denies access. For smaller organizations in particular, this approach would be feasible due to the limited number of user roles within the organization. But the shift to cloud data storage and compute – and with it, the availability of data – took off, creating more users who required faster access to more data.
The scale of modern cloud data architectures is simply too large to write exhaustive deterministic policies for each and every possible edge case. This is exacerbated by the introduction of new AI and ML tools that require access to vast amounts of data and are meant to service a wider variety of users. How are you expected to meet your growing data security needs in a timely and secure manner?
The Shift from Deterministic to Risk-Aware Security
To scale in the cloud, you need to separate policy logic from compute fabric. No team wants – or can even realistically handle – the burden of creating and maintaining platform-specific policies across every single one of your storage, compute, BI, AI, and ML platforms. However, you still need to embed security controls across your data stack in order to maintain effective cloud data governance and privacy. How can we address this paradox?
The answer, according to Carroll, is to shift philosophically and technologically away from deterministic methods and towards probabilistic, risk-aware controls.
“We’re moving from a deterministic world where we codify every potential use case, every potential end user, and what they could see, to a risk-based world where we have to make the best decision based on the context that we have in front of us,” said Carroll.
This is less about having an exact policy for every potential scenario, and more about ensuring that each query can be met with a risk-aware, context-informed access decision.
What is Risk-Aware Security?
Risk-aware controls incorporate context about data and data users so that access decisions on a given query are nuanced and highly accurate. To apply these controls and ensure your team understands the data residing in your ecosystem, you need to first consistently discover and classify sensitive data.
Then, using methods like attribute-based access control (ABAC) and policy-based access control (PBAC), you’re able to build policies that determine access based on factors related to the user, data object, environment, and usage purpose. This provides additional context on which to base access decisions, allowing for more flexibility and scalability in privacy and governance control enforcement.
“When I think of the data security space, I think of a zero trust concept where you have to look at who the user is, the query they’re running, and the data you have, and you have to make a risk-based decision,” said Carroll. “It’s not a binary authentication, [where] you either get all of it or none of it based on the app you logged into. It’s all about the authorization – that user, the data – you combine the two with the query, and you make the best risk decision you can.”
It's all about the authorization – that user, the data – you combine the two with the query, and you make the best risk decision you can.”
Matthew Carroll, Co-Founder & CEO, Immuta![]()
By understanding the context in which data is accessed, these policies ensure that security measures are both stringent and adaptable, providing an effective defense against unauthorized access while facilitating legitimate data use.
This contextual information is also crucial for enabling additional security controls, including more effective continuous security monitoring, unified data access auditing, and more. All of these controls help your team mitigate risk and maintain security, without impeding legitimate access and use.
Real-World Applications of Risk-Aware Security
To examine how real enterprises are adopting risk-aware governance and security, Carroll discussed customers from across industries. These examples included:
Pharmaceuticals
Carroll cited Roche Diagnostics as a pharmaceutical organization that recognized the potential of risk- and context-aware controls in building an innovative data architecture. While creating a data mesh architecture to decentralize data usage and controls across various teams, Roche ensured that specific domains and data products were assigned to relevant data owners.
[Read More] Enabling Data Mesh Principles for Organizational Agility
By placing governance responsibilities in the hands of those most aligned with the data, Roche ensured that controls were informed and maintained by those with firsthand context and experience, not centralized teams divorced from the data. This model of federated governance enables Roche’s teams to carry out business-critical research and development without incurring excessive risk, and has led to the development of 200+ new data products and over $50 million in benefits.
Automotives
In the automotive industry, Carroll cited the Mercedes Benz team’s handling of telemetry data as a modern use case that requires context-informed controls. The manufacturer collects vast amounts of this data from its vehicles, and needs to ensure that it is accessible to analysts around the world.
To ensure that access across markets is secure and aligned with relevant compliance laws and regulations, the Mercedes Benz team needs to be able to make informed decisions on queries without having to individually create and apply a policy for every single use case. Context-aware controls enforce these access decisions across global markets.
Financial Services
Carroll shared that massive financial services institutions like Morgan Stanley often implement governance models that require oversight from a large number of subject matter experts (SMEs). The number of people involved frequently delays access decisions, dramatically slowing time-to-data for analysts who need to mine timely insights.
To solve this problem, financial institutions are beginning to consider distributed architectures like the data mesh, which facilitate more accessible domain-based data storage, access, and use. As with Roche’s experience, these distributed architectures align controls with informed teams, and enable faster access without sacrificing security.
Media & Entertainment
Across media and entertainment companies, Carroll noted a widespread shift towards increasingly personalized marketing on a global scale. With more targeted marketing comes a slew of concerns around individuals’ privacy, as marketing initiatives should in no way endanger users’ personally identifiable information (PII).
To prevent this added risk, media organizations need dynamic risk-aware controls that incorporate data sovereignty and privacy considerations into access determination across global markets. When supplemented by privacy-enhancing technologies (PETs) like k-anonymization and differential privacy, these controls enforce proactive security to protect data subjects and maintain regulatory compliance. When applied effectively, these controls ensure that no marketing user’s query will turn up data that they should not see.
[Read More]How One of the World’s Top Streaming Services Scaled Data Access
Leveraging Artificial Intelligence for Risk-Aware Security
While incorporating AI and machine learning tools contributes to modern data stack complexity, they also provide exciting opportunities to enhance risk-aware controls. In a recent AI Security & Governance Report, 80% of survey respondents agreed their organization is capable of identifying and mitigating threats in AI systems. Respondents also identified a number of opportunities for incorporating AI tools into their security initiatives, including anomaly detection, security app development, phishing attack identification, and enhanced incident response.
“We have to also rethink how we’re tagging our data relative to risk,” said Carroll. “That’s an area where we’ve started to introduce AI within our platform, to make that far more intelligent and far more business-centric than generalized.”
By introducing AI-driven capabilities into dynamic security platforms – like the Immuta Data Security Platform – more streamlined contextual information will inform policy decisions. These powerful models help remove manual access request and approval efforts, and support context-based decision making.
What we have to do is look at all the metadata and leverage AI to derive what's the best approach to managing by exception.”
Matthew Carroll, Co-Founder & CEO, Immuta![]()
Tools like large language models (LLMs) also provide context for your team’s data users, helping them understand why they are granted or denied access to certain data.
“What we have to do is look at all the metadata and leverage AI to derive what’s the best approach to managing by exception,” said Carroll. “A user should always be able to ask a question of the data – they may get zero results, because they shouldn’t have it. But then again, it’s leveraging artificial intelligence to say ‘Ok, I’ll give you more, but here’s why we gave you more.’”
The Future of Risk-Aware Policy Making in Data Security
As we navigate the complexities of the evolving cloud data ecosystem, the shift towards risk-aware policy making is a pivotal evolution in data security. This approach not only brings additional intelligence and adaptability to protective protocols, but also ensures that any access decision is responsive to the real-time context in which it occurs.
Whether in the pharmaceutical, automotive, financial services, media, or any other industry, there is a strong case to be made for incorporating risk- and context-aware data security into your data ecosystem – and now is the time to do so, as AI and decentralization take center stage.
Leveraging sensitive data discovery, dynamic data access controls, and continuous security monitoring – and incorporating AI tools where possible – enables your team to maintain robust security and compliance while streamlining data access across user groups, geographies, and more.
For more insights, watch Matt Carroll and Sanjeev Mohan’s full discussion here. If you’d like to learn more about incorporating risk-aware data security into your data stack, request a demo with our team.
Start leveraging risk-aware data security.
Get in touch with our team.