By the time COVID-19 was classified as a pandemic, it was already spreading like wildfire. As healthcare professionals, scientists, and federal agencies tried to understand the nature of the virus and implement appropriate mitigation measures and public health guidelines, it was quickly seeping into all corners of the globe.
As with any fast-moving public health threat, a timely response was paramount to avoiding a worst case scenario. Yet, unlike other threats like the flu and natural disasters, there is no precedent for COVID-19. This means researchers are critical to informing appropriate responses — and they need real time access to sensitive data for analytics.
The Center for New Data established its COVID Alliance program in response to this urgent need. The nonprofit is focused on accelerating research on new and novel data to provide valuable insights that can help ethically solve public problems like the pandemic. But to empower their data scientists and academic researchers to analyze and collaborate on projects involving sensitive data, the Center needed an all-in-one solution that wouldn’t require additional overhead, tools, or most importantly, time.
Monitoring Superspreader Events & Sensitive Data Analytics
The pandemic necessitated and normalized a number of actions that would have seemed out of the ordinary just a year earlier, like social distancing, mask wearing, and contact tracing. But monitoring the movements of populations was of particular importance to The Center for New Data’s researchers. To track and predict community spread, it was critical to know where groups of people were when they may have contracted COVID-19, and more importantly, where they subsequently traveled.
In August of 2020, 500,000 people traveled from multiple states to the annual Sturgis Motorcycle Rally in Sturgis, South Dakota. In a time when even small gatherings were prohibited in many states, it was easy to pinpoint the rally as a potential superspreader event. The Center for New Data was able to identify and aggregate the anonymized geolocation data from 11,000 people who traveled to Sturgis. But without a secure data access governance layer in its cloud data ecosystem, this data would have been useless. Similarly, without the proper de-identification measures in place, the follow-up analysis of another superspreader event in Lake of the Ozarks and even larger dataset of 50 million devices procured by The Center for New Data covering the 2020 Thanksgiving weekend would have sat idle — while potential virus spread remained unpredictable.
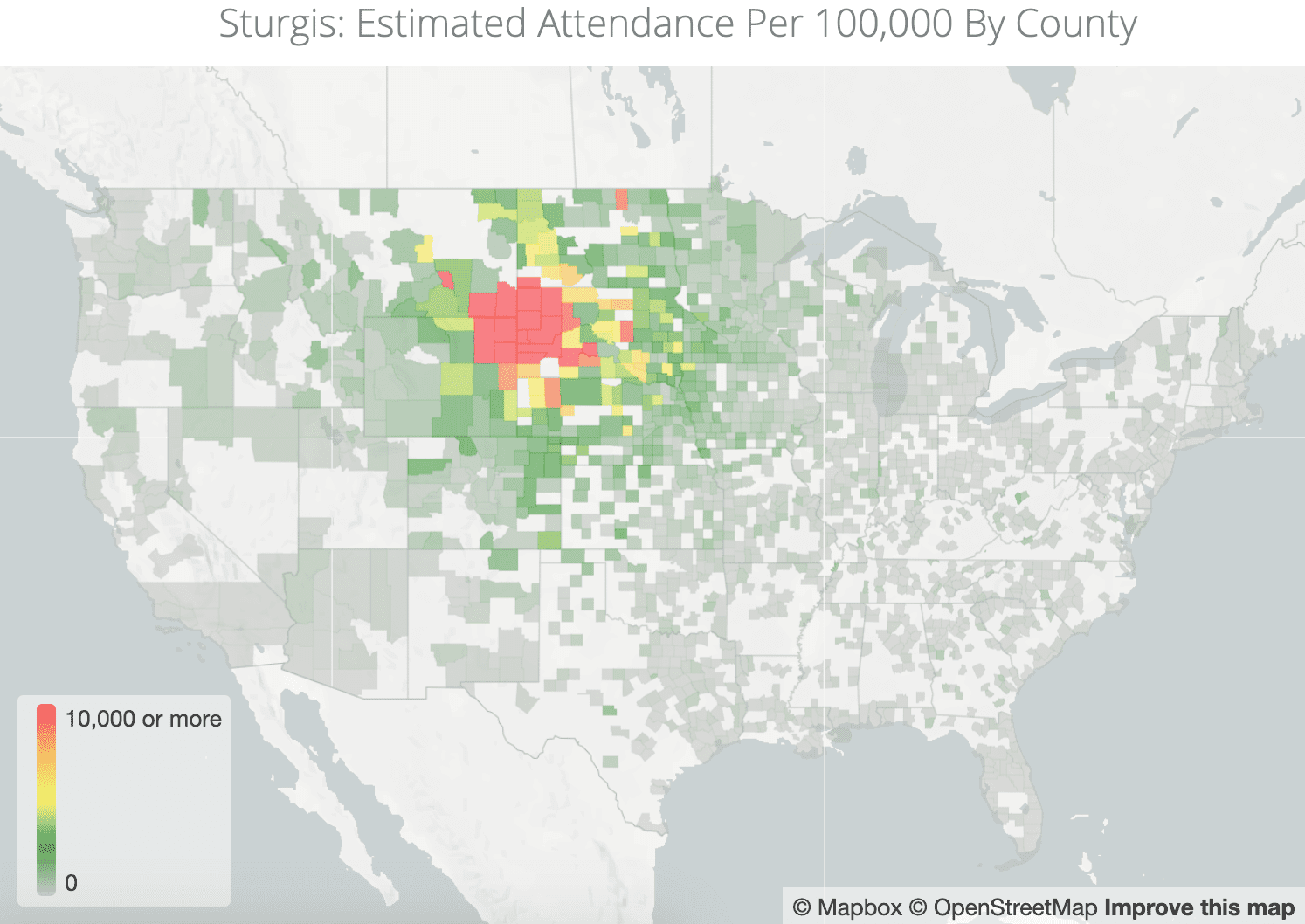
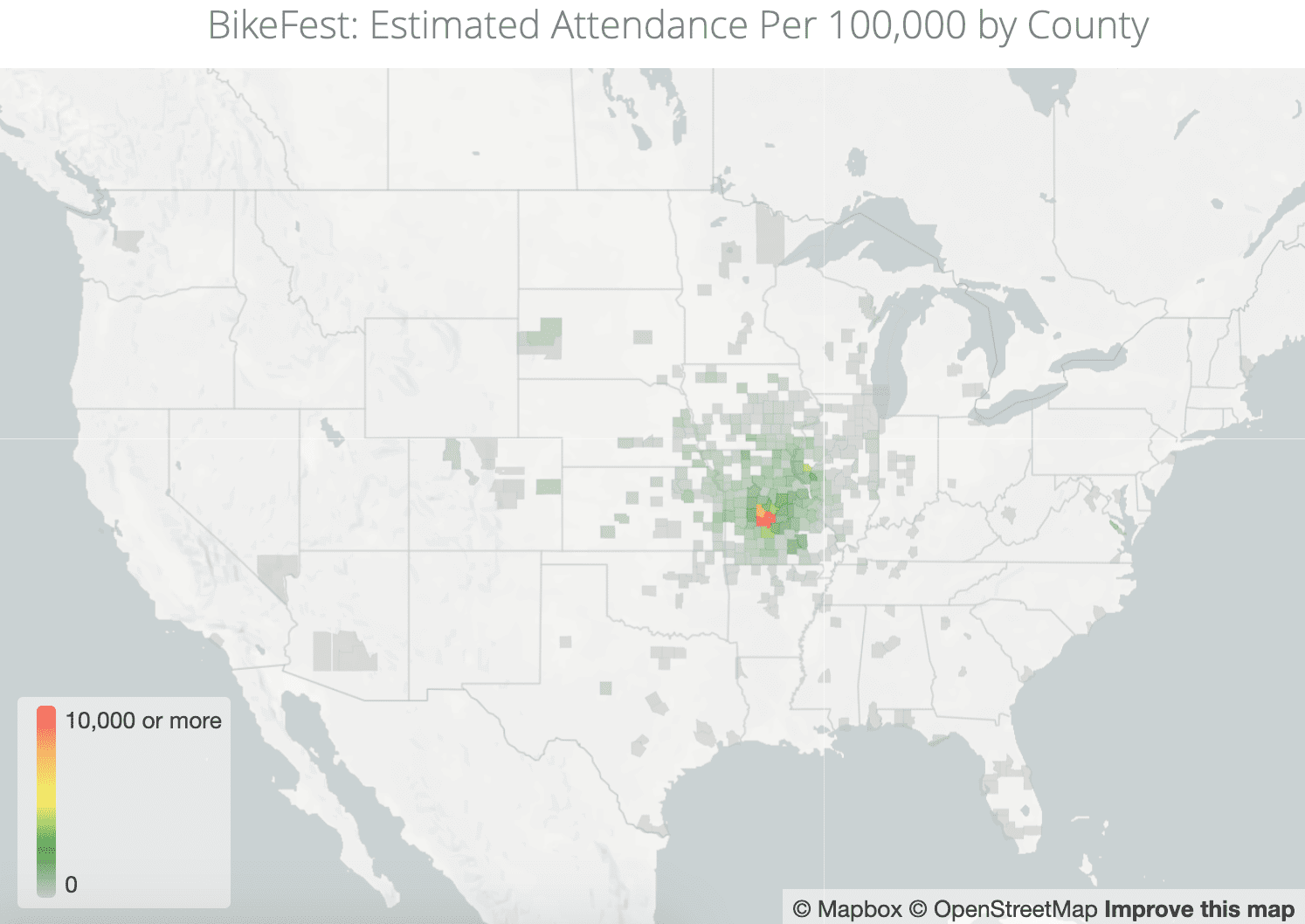
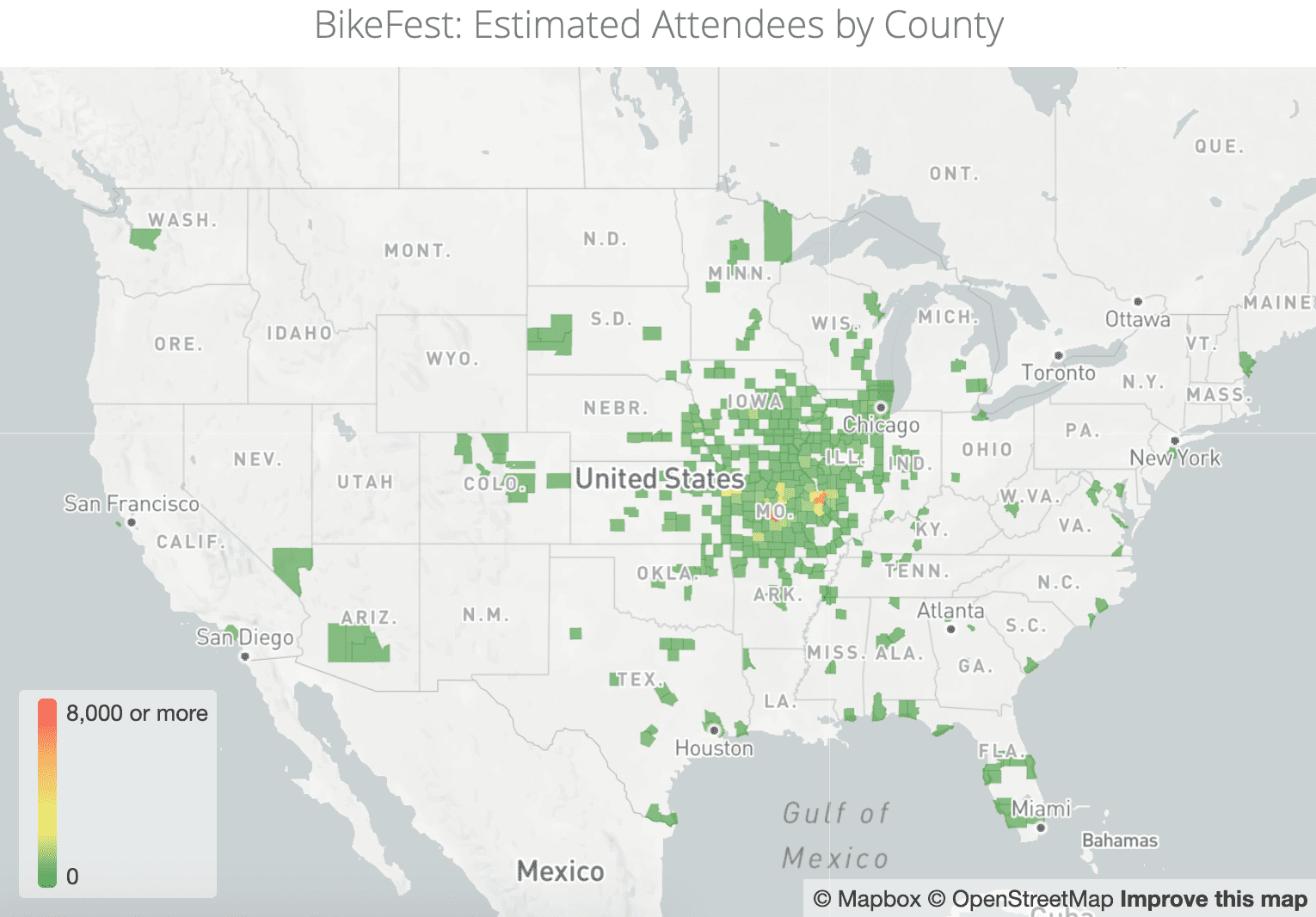
Since sensitive personal data is notoriously vulnerable to re-identification — as demonstrated by Latanya Sweeney’s work proving 87% of the population can be identified by birthdate, gender, and zip code — The Center for New Data needed a way to meet its legal, ethical, and contractual guidelines for protecting it soundly without sacrificing utility. To complicate matters, The Center for New Data relied upon data professionals and researchers with varying backgrounds and vastly different data access needs, so enabling collaborative data analysis without inadvertently granting unauthorized data access was a necessary obstacle to overcome.
Enabling Real Time Analysis on Sensitive Data
The Center for New Data needed to maximize data privacy and utility quickly and securely. The initial approach of reviewing individual research proposals and creating new data access controls in Snowflake for each of them was burdensome and inefficient. The Center needed a self-service data access process in which data engineers managed and established analytics environments for researchers.
With COVID-19 showing no signs of slowing, The Center for New Data incorporated Immuta into its data environment. Immuta’s native integration with Snowflake streamlined a secure data preparation process that democratized data access across The Center’s population of researchers. Now, researchers are better able to analyze sensitive data in real time with Immuta’s:
- Active data catalog, which enables self-service workflows through which researchers can request data access, approve usage purposes, request access control changes, and propose new projects.
- Sensitive data discovery, which automatically classifies and tags direct identifiers, indirect identifiers, and other sensitive information so the proper rules and policies can be efficiently applied.
- Fine-grained, attribute-based access controls, which ensure researchers have the right access to the right data at the right time, while avoiding data copying and role explosion.
- Dynamic data masking, including privacy enhancing technologies (PETs) like k-anonymization, differential privacy, and format-preserving masking, which provide comprehensive protection against re-identification.
“The ability for us to manage access controls, deploy privacy enhancing technologies, and rapidly implement novel frameworks of governance for our research teams has been a breath of fresh air, with no management or overhead costs for adding additional cloud database solutions,” said Ryan Naughton, co-executive director at The Center for New Data.
With these features in place, not only were The Center for New Data’s researchers able to quickly, securely, and compliantly analyze sensitive geolocation data from superspreader events and Thanksgiving holiday travel, which helped guide public health guidelines in several states, but The Center itself was able to save more than $1 million in annual data engineering costs by automating manual, burdensome, and error-prone processes. Data engineers and architects were able to decrease both the number of access control policies and time to data access, becoming more productive and efficient in the process.
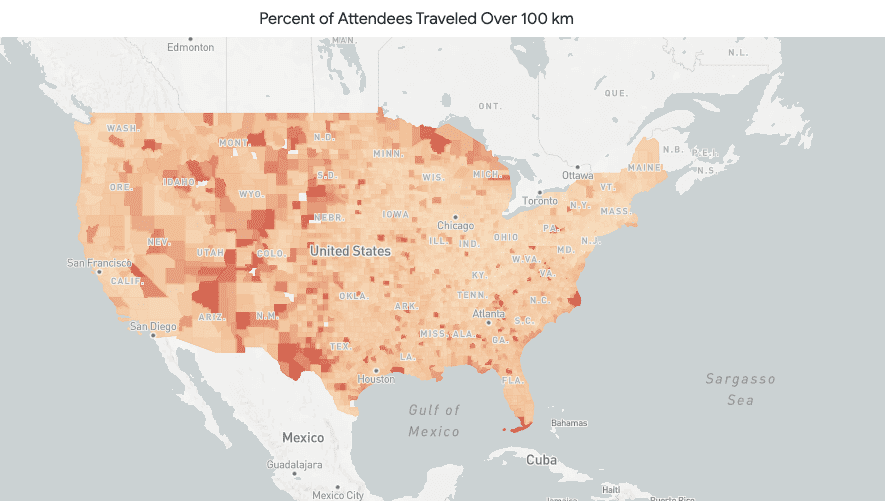
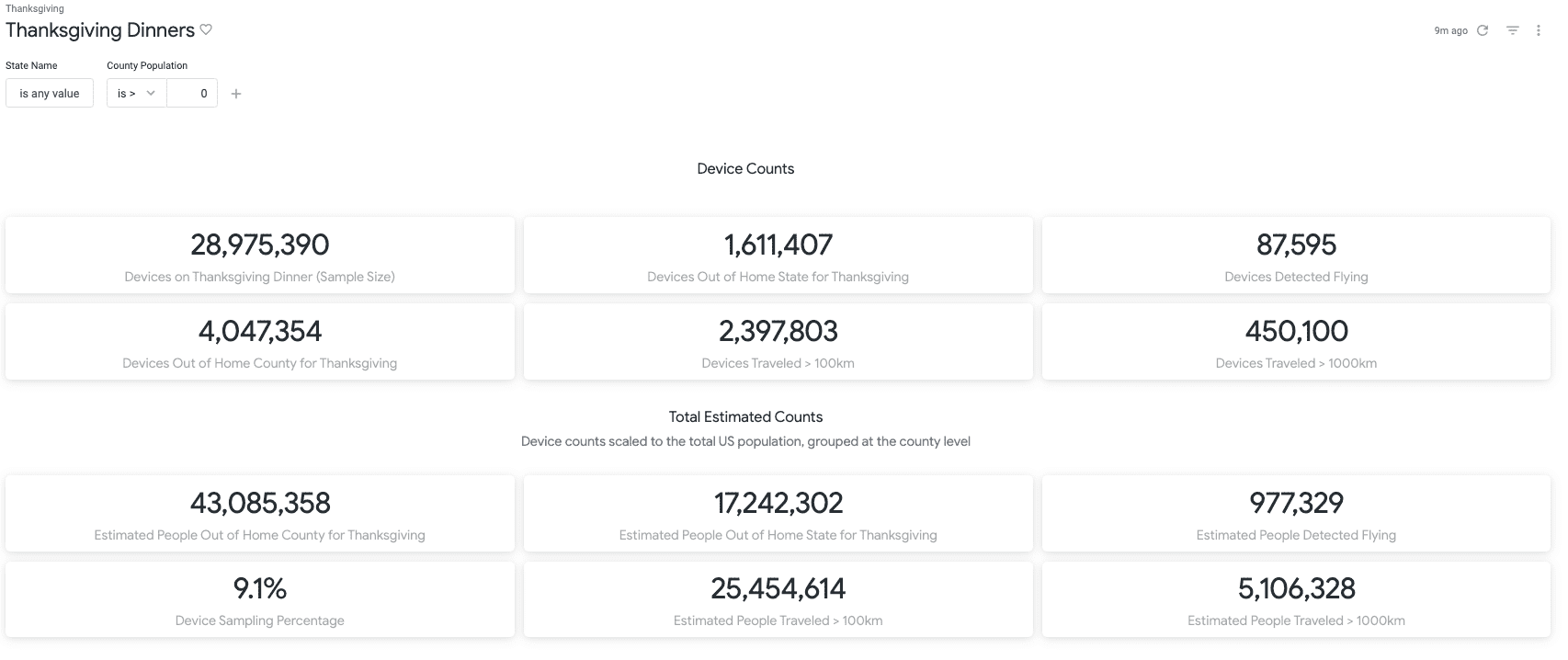
To learn more about how The Center for New Data maximized the value of its sensitive data without sacrificing security, and download the case study here.