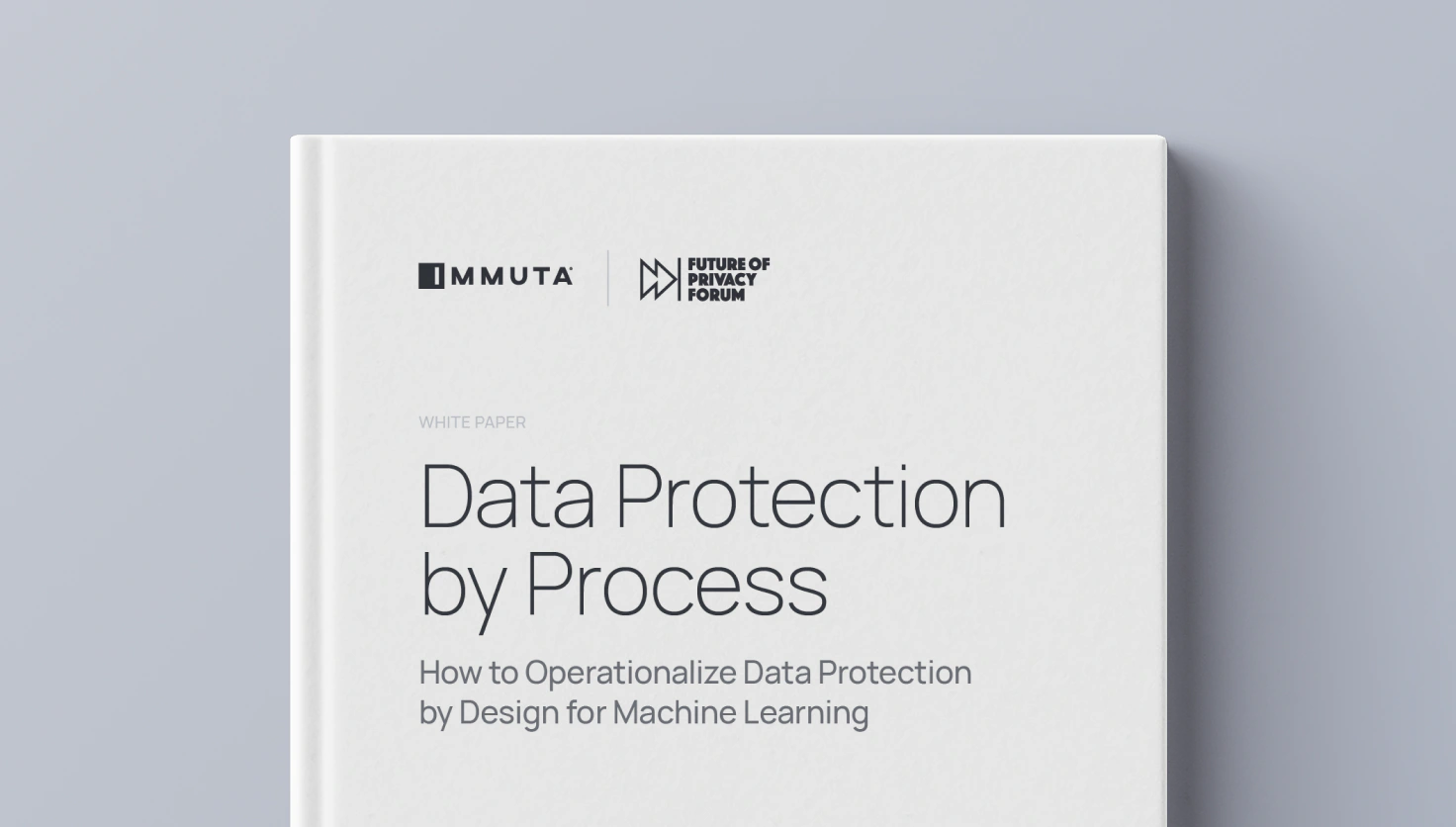
“The GDPR has been criticized by many for being too high-level or outdated and therefore impossible to implement in practice. Our work aims to bridge the gap between theory and practice, to make it possible for data scientists to seriously take into account data protection and privacy requirements as early as possible. Working closely with engineers, we have built a framework to operationalize data protection by design, which should be seen by all as the backbone of the GDPR.”
Data protection by design is the principle of integrating privacy and data security measures into the development of systems, products, and processes from the outset – instead of treating them as an afterthought. By proactively incorporating privacy features and controls, organizations aim to abide by compliance laws and regulations, build trust, and mitigate risks associated with processing personal information.
This white paper, released in partnership with the Future of Privacy Forum (FPF), provides guidance on embedding data protection principles within the life cycle of a machine learning model, as well as clear instructions on how to fulfill the Data Protection by Design (DPbD) obligation. You’ll find out from a team of researchers how to build a DPbD strategy in line with data protection principles.
Authors:
Sophie Stalla-Bourdillon, Immuta
Alfred Rossi, Immuta
Gabriela Zanfir-Fortuna, FPF